
-
-
-
-
URL copied!
As human beings, we want to understand what the future holds for us. We make decisions based on the information available to ensure all risks are either resolved or mitigated. Often, these decisions are based on analysis done on historical data.
Consider this: I plan to run a marathon two months from now. I have never run a marathon – just a couple of 10Ks. I need a plan to prepare myself.
What is the data available to me right now to make this plan? It comes from the preparations I did for my 10K. I did not start running 10K on my first day; I started with 2K. After 10 Days, I was able to take it up a notch to 4K, and a week after that to 5K. It took me a month and a half to practice for my first 10K.
Weather also played a part in my runs. On a humid morning, I could not do a 5K so easily. Cool nights were better. But to make a long story short, I had enough data from my past experiences to begin preparations for the marathon.
Now that I have the data, can I predict my success or failure for the marathon? I still have to create key milestones and KPIs by which to measure my performance. If the marathon is in two months, I should be able to hit 10K in the first three weeks.
Am I in shape to start running 5K to start with? What about the weather? How about where I plan to train? Also, do I have enough time in my schedule to plan for my training?
Similar questions arise in the lifecycle of the products we are building. Leadership – both at the team and program level – need data and information to help remove impediments and bring predictability. These can be driven by two different kinds of indicators: leading and lagging.
Leading Indicators
Leading indicators focus on the inputs going into building a product. These help determine whether we have the right ingredients in place to build the right product in a timely manner.
Leading indicators are hard to measure and a lot of times depend on historical data available at a point in time. In the story above, the leading indicators for me to run a marathon successfully are: How much time will I be putting in everyday to train for the run?
For example, if I say two hours, I can track that on a daily basis and see if I am doing that because if not, I will surely miss my target. In product development, the health of a backlog is a good indicator of where my teams are heading before the start of a sprint or PI. Some other leading indicators can be:
- Release Burndown used to track our progress for a release. This will help programs make the right decisions.
- Backlog Readiness, as the health of our backlog is a key leading indicator of how our Sprints and PI will go.
- Epic Readiness.
- Story Readiness.
- Capacity v/s Load. Do we have enough work to feed our teams and/or trains?
- Stable Velocity. Based on historical data, will our teams be able to achieve the commitments they are making?
Lagging Indicators
Lagging indicators focus on the outcome. They are easy to measure and help develop a pattern of how teams and programs are doing. Leaders can gain insight from this data, do root cause analysis, and find where the bottlenecks might be or what areas to improve.
In my story above, after two weeks of training, reviewing my performance data gave me insight into how well prepared my body was for the run. Based on that insight, I made changes to my routines to ensure I would improve on them in the next two weeks. Lagging indicators can be:
- UAT/Production Defects Density, the quality of the code deployed in Production/Higher controlled environments.
- The number of unplanned Production Releases. How often are we deploying code in production? RCA will lead to key areas to fix.
- Sprint/PI Spillovers (Committed v/s Completed), or the team’s ability to meet the commitments.
- Sprint Churn. How stable are our backlogs during the Sprints?
These examples of leading and lagging indicators vary from program to program and based on the point in time or milestone for which you are developing these reports. They can also be different for various leadership groups and the structural hierarchy from which you are looking at product development.
Ultimately, you want your program to define leading and lagging indicators for tracking its health. And last but not least, the data used for building these metrics should come from a single source of truth.
Have you defined your leading and lagging Indicators yet?
Top Insights
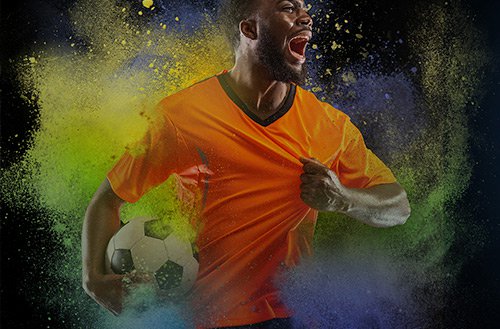
Manchester City Scores Big with GlobalLogic
AI and MLBig Data & AnalyticsCloudDigital TransformationExperience DesignMobilitySecurityMedia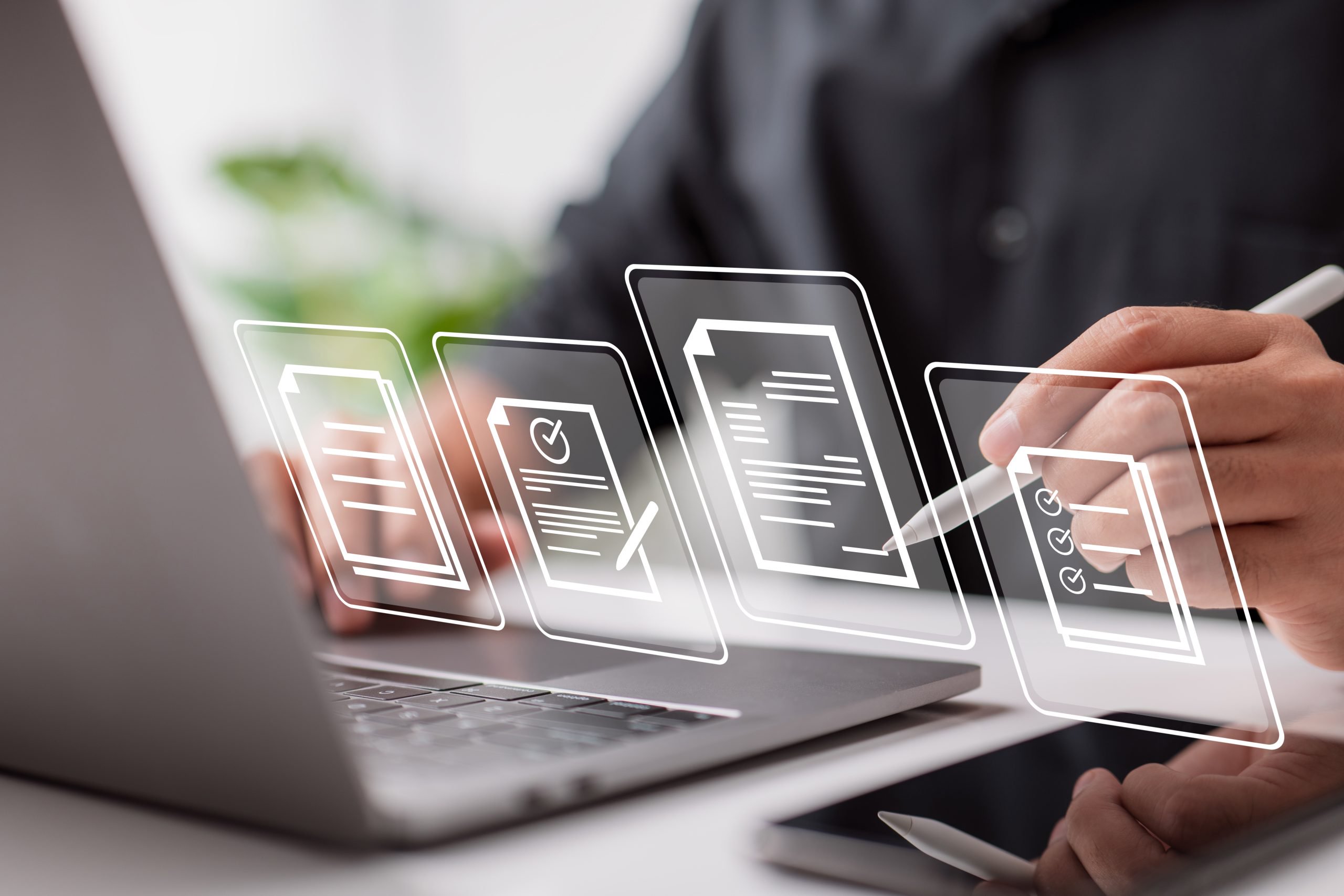
Twitter users urged to trigger SARs against energy...
Big Data & AnalyticsDigital TransformationInnovation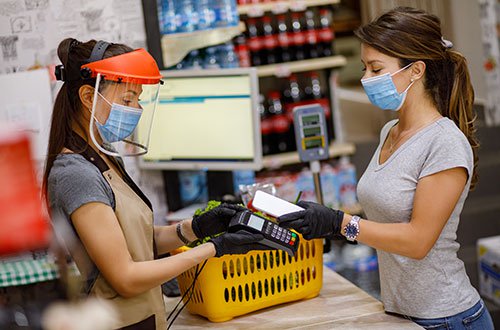
Retail After COVID-19: How Innovation is Powering the...
Digital TransformationInsightsConsumer and RetailTop Insights Categories

Let’s Work Together
Related Content
GenAI in Action: Lessons from Industry Leaders on Driving Real ROI
Generative AI (GenAI) has the potential to transform industries, yet many companies are still struggling to move from experimentation to real business impact. The hype is everywhere, but the real challenge is figuring out where AI can drive measurable value—and how to overcome the barriers to adoption. In the latest episode of Hitachi ActionTalks: GenAI, … Continue reading Predicting Program Health – Insights into Leading and Lagging Indicators →
Learn More
Unlock the Power of the Intelligent Healthcare Ecosystem
Welcome to the future of healthcare The healthcare industry is on the cusp of a revolutionary transformation. As we move beyond digital connectivity and data integration, the next decade will be defined by the emergence of the Intelligent Healthcare Ecosystem. This is more than a technological shift—it's a fundamental change in how we deliver, experience, … Continue reading Predicting Program Health – Insights into Leading and Lagging Indicators →
Learn More
GlobalLogic wins at the 2023 Analytics Institute Awards, Dublin
*This blog was updated on Friday 16th June. The team is excited to announce that GlobalLogic was named winners of the Emerging Technology Award at last night's Analytics Institute Awards! This prestigious award recognises organisations that have successfully employed new technologies such as IoT, Edge Computing, Machine Learning, or RPA. Our submission showcased the successful application of … Continue reading Predicting Program Health – Insights into Leading and Lagging Indicators →
Learn More
MLOps Principles Part Two: Model Bias and Fairness
Welcome back to the second instalment of our two-part series – MLOps (Machine Learning Operations) Principles. If you missed part one, which focused on the importance of model monitoring, it can be found here. This blog explores the various forms that model bias can take, whilst delving into the challenges of detecting and mitigating bias, … Continue reading Predicting Program Health – Insights into Leading and Lagging Indicators →
Learn More
The GlobalLogic Academy Programme – a personal, introspective recollection
Ben Graham – Academy 2022 Graduate/Delivery Consultant I am currently in the DevOps capability for consulting and a recent graduate of the Academy 2022 programme which ran from September to December. I’d like to detail my thoughts on the process and share how my fellow graduates and I felt going on this journey. The GlobalLogic … Continue reading Predicting Program Health – Insights into Leading and Lagging Indicators →
Learn More
Seven steps to break down systemic gender barriers
Despite progress over the years, women are still significantly underrepresented in tech. Systemic gender barriers – such as unconscious bias, lack of access to education, and cultural norms – can make it difficult for women to break into the tech industry. But how do we break down these barriers? Follow our simple step by step … Continue reading Predicting Program Health – Insights into Leading and Lagging Indicators →
Learn More
Share this page:
-
-
-
-
URL copied!