
-
-
-
-
URL copied!
Artificial intelligence (AI) has been hailed as the new electricity due to its potential to transform business. Unfortunately, unleashing the full potential of AI is a challenge for many enterprises.
It’s proving to be a struggle to get the machine learning (ML) models underpinning new AI applications over the line. Despite widespread ML investment by businesses that understand the potential of this technology, a staggering 60% of ML projects initiated never make it into production. To put this into perspective, imagine a car manufacturer that has a budget to build 100 cars on its production line but only completes 40 cars. This is obviously an undesirable outcome and an unsustainable business model.
Once developed, problems persist. Gartner predicts: “through 2020, 80% of AI projects will remain alchemy, run by wizards whose talents will not scale in the organisation.”
One issue is that the growth and evolution of data science tools and technologies and expertise has not been supported with equally mature ML development methods or processes. This has a direct impact on the ability to effectively manage release cycles to deploy ML models in production and manage the continuous retraining and release cycles required. The remains a major barrier to the success of ML projects for most organisations.
If this sounds alarmingly familiar, don’t despair.
The emergence of MLOps – a practice for collaboration and communication between data scientists and operations teams to help manage ML development lifecycles – is a game changer.
At GlobalLogic, our MLOps service blends our deep expertise in enterprise-grade DevOps with our pedigree in IT operations service and support and ML engineering capabilities. Importantly, we help you clear not just the technical hurdles but the cultural ones too.
Our MLOps frameworks are built on Amazon SageMaker to simplify and automate ML workflows and get models into production faster and more cost effectively.
Interestingly, several enhancements were announced this month at AWS re:Invent that will bring even more workflow efficiencies. One is Amazon SageMaker Studio, an integrated development environment (IDE) that allows developers and data scientists to build, code, develop, train and tune machine learning workflows using a single interface.
Another announcement that caught my eye is Amazon SageMaker Model Monitor. It detects deviations such as data drift which degrade model performance over time, so you can take remedial action.
Amazon Sagemaker Operations for Kubernetes is yet another new and exciting capability. It enables organisations to release workflows with much-needed portability and standardisation, supporting security, high availability and regulatory requirements.
Around our MLOps frameworks we wrap our unique, tried and tested pod-style approach to enablement. Our MLOps Enablement Pods provide outcome-focused teams of data scientists and MLOps engineers that remain flexible in their resource profiling so that your day-to-day team is exactly what you need at every stage.
Specifically, our MLOps Enablement Pods operate in sprints, embedding in your teams for short periods to instil new skills and tooling as well as new processes and ways of working into your business, while keeping a tight control on costs. By keeping your pipelines clear, we help you get AI projects into production quickly and cost effectively.
To find out how our MLOps wizardry can help you extract more value from your ML strategy and unblock your AI project pipelines, please get in touch with our team here.
Top Insights
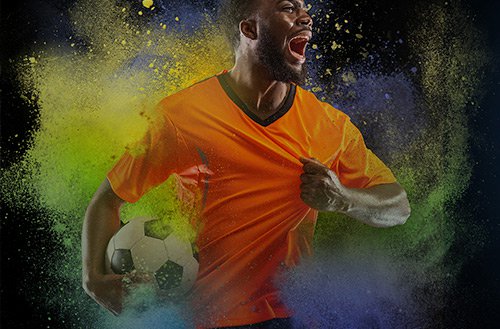
Manchester City Scores Big with GlobalLogic
AI and MLBig Data & AnalyticsCloudDigital TransformationExperience DesignMobilitySecurityMedia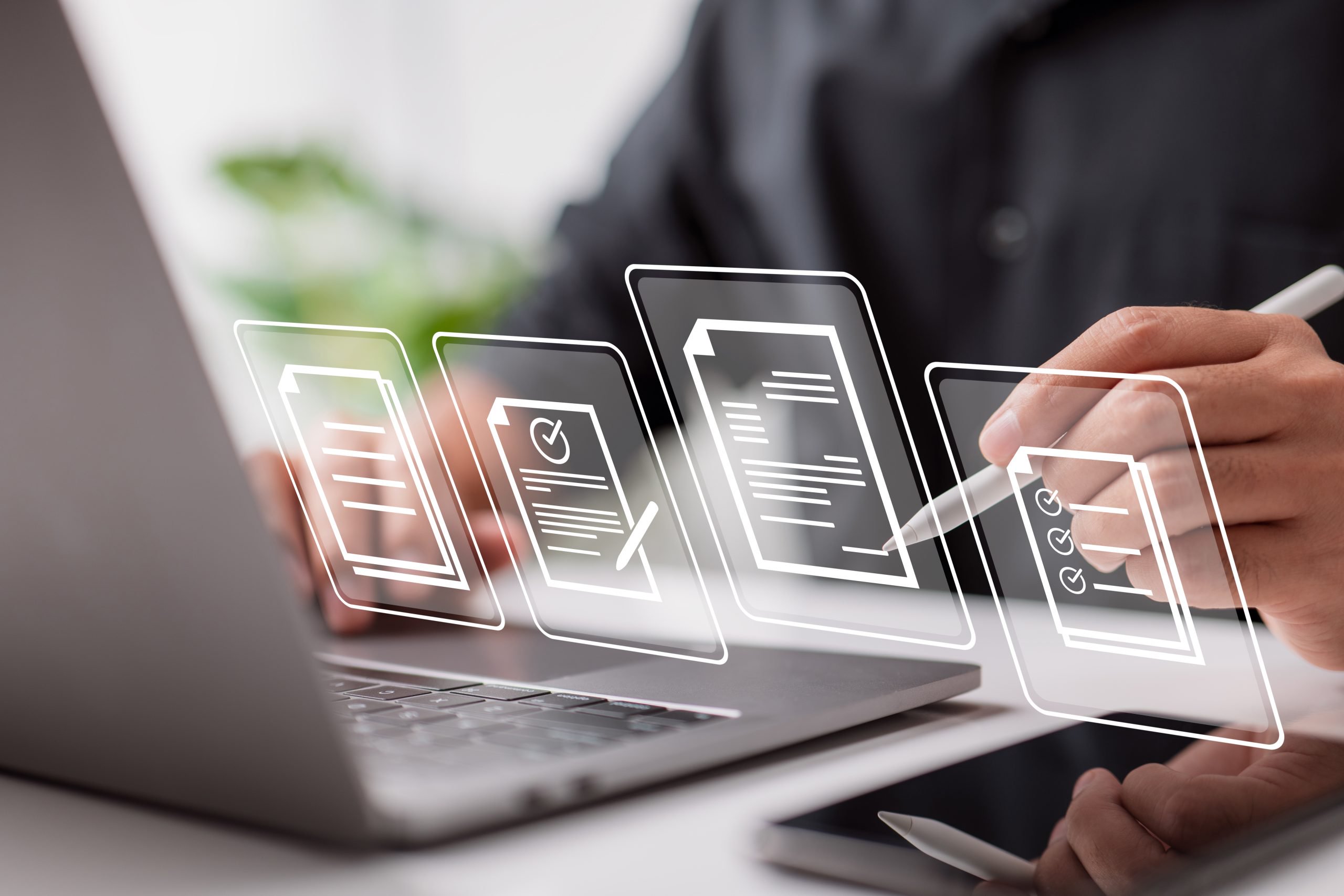
Twitter users urged to trigger SARs against energy...
Big Data & AnalyticsDigital TransformationInnovation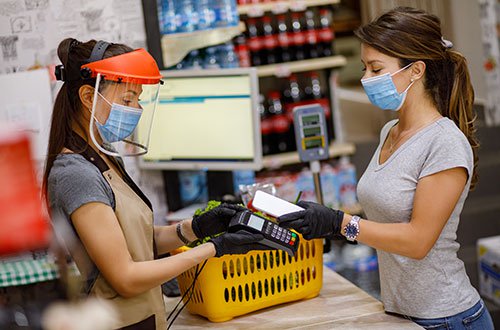
Retail After COVID-19: How Innovation is Powering the...
Digital TransformationInsightsConsumer and RetailTop Insights Categories

Let’s Work Together
Related Content
The Rise of The Invisible Bank
Banks will power experiences, but everyone will ignore them. Inspiration for this blog title comes from Jerry Neumann, the author of the blog Reaction Wheel, who wrote in 2015 that ‘software eats the world and everybody ignores it’. Neumann also observed that ‘information and communications technology becomes ubiquitous but invisible’ – in other words, … Continue reading Powering Up MLOps Production Lines for Frictionless AI →
Learn More
MLOps Principles Part Two: Model Bias and Fairness
Welcome back to the second instalment of our two-part series – MLOps (Machine Learning Operations) Principles. If you missed part one, which focused on the importance of model monitoring, it can be found here. This blog explores the various forms that model bias can take, whilst delving into the challenges of detecting and mitigating bias, … Continue reading Powering Up MLOps Production Lines for Frictionless AI →
Learn More
How Smart Cars Will Change Cityscapes
Which technologies do we need to improve the quality of life for people in the city and make governance more effective? What role does a car play in the city of the future, and how can it make traffic safer? GlobalLogic’s engineers are already working on solving these issues.
Learn More
What happens in Vegas stays in Vegas
At GlobalLogic, both our consultants and clients love utilising Amazon Web Services (AWS) technologies extensively. This passion has helped us secure a number of highly coveted accreditations, including Amazon Connect Service Delivery Partner, AWS DevOps Competency Partner and AWS Advanced Consulting Partner. As part of our partnership, we thoroughly enjoy attending AWS events to keep … Continue reading Powering Up MLOps Production Lines for Frictionless AI →
Learn More
Share this page:
-
-
-
-
URL copied!