
-
-
-
-
URL copied!
Machine Learning (ML) has been hailed as the new electricity due to its potential to transform business. There is an increasing recognition from businesses that ML could help them reduce costs, increase efficiencies, fuel innovation, unleash opportunity, and deliver value in many guises – both within organisations and out to customers. In fact, M&S gave a public talk around Data Science and the development and productionisation of 11 ML models, noting these models delivered £70 million in incremental revenue.
Learning how to apply and harness ML can create a huge competitive advantage. And with that promise, we’ve seen many businesses investigate and delve deeper into ML.
Despite widespread ML investment by businesses, a staggering 85% of ML projects never make it into production (Gartner). In other words, the vast majority of ML projects never realise their value as they remain a half-baked idea within a siloed team. This happens for a number of reasons. The first is that there is no guarantee an ML use case will result in a reliable ML model. This can be exacerbated by common difficulties such as a lack of communication with the stakeholders, lack of experience in real case scenarios and lack of using the right tools. Businesses also struggle to reach the right scale to consistently deliver the outcomes and expected value from ML projects – trends expected to persist in 2022.
The ‘Industrialisation of ML’
AWS will often talk about the ‘Industrialisation of ML’ as having three major components with data at the centre:
- ML Infrastructure
- ML Tools
- MLOps Practices
With a great deal of flexibility afforded, it means the approach to ML model development and deployment can be varied. This involves taking account of your business’s expertise in-house, the maturity of the ecosystem for ML development, the use cases for ML and subsequent outcomes you want to achieve – in other words, what success looks like for you.
With the AWS ML stack (AI Services, ML Services and ML Frameworks & Infrastructure), you can use pre-packaged AI services, pre-trained models and even AutoML that trains an ML model with one click. These are a great starting point but can be quite generic. If you would benefit from complex and bespoke model requirements – quite often the case for Financial Services – then AWS SageMaker and Data Science are a powerful combination with huge extensibility.
There are other infrastructure considerations too, prompted by the requirements for successful ML projects.
The challenge of data quality
Machine Learning projects use large datasets. More data will lead to better predictions. But as the volume of data increases, so do the challenges. Good data management based on a modern data platform – preferably cloud-native – is a key consideration.
The implementation and use of DataLakes and Data Clouds to facilitate the unification of data from 1st and 3rd party sources, is a desired step ahead of making sure your data is suitable for ML opportunities. A sticky affair for most, although digitally native organisations might find it easier to clear some of the hurdles early on. At GlobalLogic, we leverage the right ecosystem to maximise the impact and simplify the task. This means unifying your data with capabilities from our AWS and Snowflake partnerships and experience.
Before we jump too far ahead, we need to take a step back and look at the data itself.
Consideration of a number of critical steps and pointed questions early on in an ML project will increase the likelihood of success, with this success moving into production and tangible impact. Let’s look at the approach and the data.
Your organisation’s data quality and the degree to which is it fit-for-purpose and suitable to support the ML use cases and business objectives is such an important foundation for success. This is where Data Science and Data Scientists make their mark.
GlobalLogic’s approach to Data Science
Here at GlobalLogic, we believe it’s important to work closely with our clients throughout the entire Data Science project lifecycle. We start by engaging with your organisation in a Value Stream Assessment (VSA) workshop. This involves understanding the business challenges, what the organisation is trying to achieve, your requirements and what data is available. We use this to identify all the opportunities and understand the limitations.
We also ensure we are aligned with your preferred outcomes by discussing the opportunities with your main stakeholders and involving the relevant business departments. Continuing success is ensured by involving stakeholders at an early stage and throughout our Data Science engagements, ensuring the solutions being developed can be embedded in their relevant departments/teams.
Next, we run an Exploratory Data Analysis (EDA) on the data available, to understand the data, determine the data quality, and confirm the feasibility of the opportunities scoped on the previous stage. We will identify new patterns and insights on this data that will provide additional value to your organisation. Additionally, we will create a plan for a Proof of Concept (PoC) continuation of the project and will transform the dataset ready for the first stages of modelling.
During the PoC phase, we create a custom ML model trained on the EDA datasets. We will refine our dataset transformations, augment, and clean the data further and, engineer relevant features. Most importantly, we demonstrate that our model solves the particular business challenge or use case identified.
To accelerate our workflows and enable us to deliver powerful Data Science projects faster, we leverage our expertise on tools such as Amazon SageMaker and QuickSight. We are also experts in Snowflake for accelerating data transformation in a highly scalable manner.
Once the desired objectives and requirements are achieved, we move to the Productionisation stage, where we take full advantage of the power of AWS’s and Snowflake’s technologies and ensure that we address major challenges with productionising ML models, such as enterprise scale infrastructure, security, regulation and support.
Working with GlobalLogic de-risks your investment in Data Science. Not only will you see tangible benefits before investing significant amounts of time and money, we will upskill your team as the project unfolds so you gain the skills you need to achieve continuous success.
Maximising MLOps
Let’s recap for a moment.
We’ve looked at the value of ML to your business and the challenges that can often be involved. We have also discussed the key components needed to industrialise your ML projects – including considerations for a modern data platform – and we have spent time focusing on the data and the impact a Data Science centric approach contributes to a successful project.
You’ve successfully moved your models into production, they’re live and in the wild and delivering insight and results. Mission accomplished!
But there is one final consideration to close the loop on that success and ensure it’s maintained. That is the role of MLOps. MLOps effectively manages release cycles, deploys ML models in production and manages the continuous retraining and release cycles required. The emergence of MLOps – a practice for collaboration and communication between Data Scientists and operations teams to help manage ML development lifecycles – is critical.
At GlobalLogic, our MLOps service blends our deep expertise in enterprise-grade DevOps with our pedigree in IT operations service and support, and our ML engineering capabilities. Importantly, we help you clear not just the technical hurdles, but any cultural and regulatory ones too.
Built on Amazon SageMaker, our MLOps frameworks have been designed to simplify, standardise and automate ML workflows and get models into production faster and more cost effectively.
Around our MLOps frameworks we wrap our custom, tried-and-tested Pod-style approach to enablement. Our MLOps Enablement Pods provide outcome-focused teams of Data Scientists and MLOps Engineers that remain flexible in their resource profiling so that your day-to-day team is exactly what you need at every stage.
To find out how our Data Science and Machine Learning experts can help you extract more value from your ML strategy and unblock your AI project pipelines, please get in touch with our team here.
Top Insights
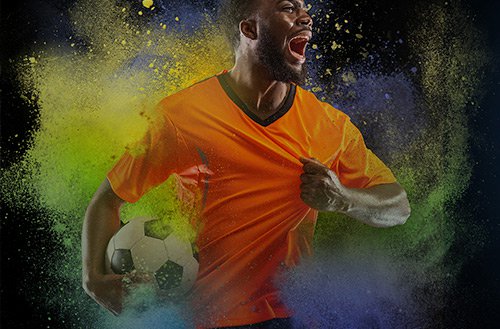
Manchester City Scores Big with GlobalLogic
AI and MLBig Data & AnalyticsCloudDigital TransformationExperience DesignMobilitySecurityMedia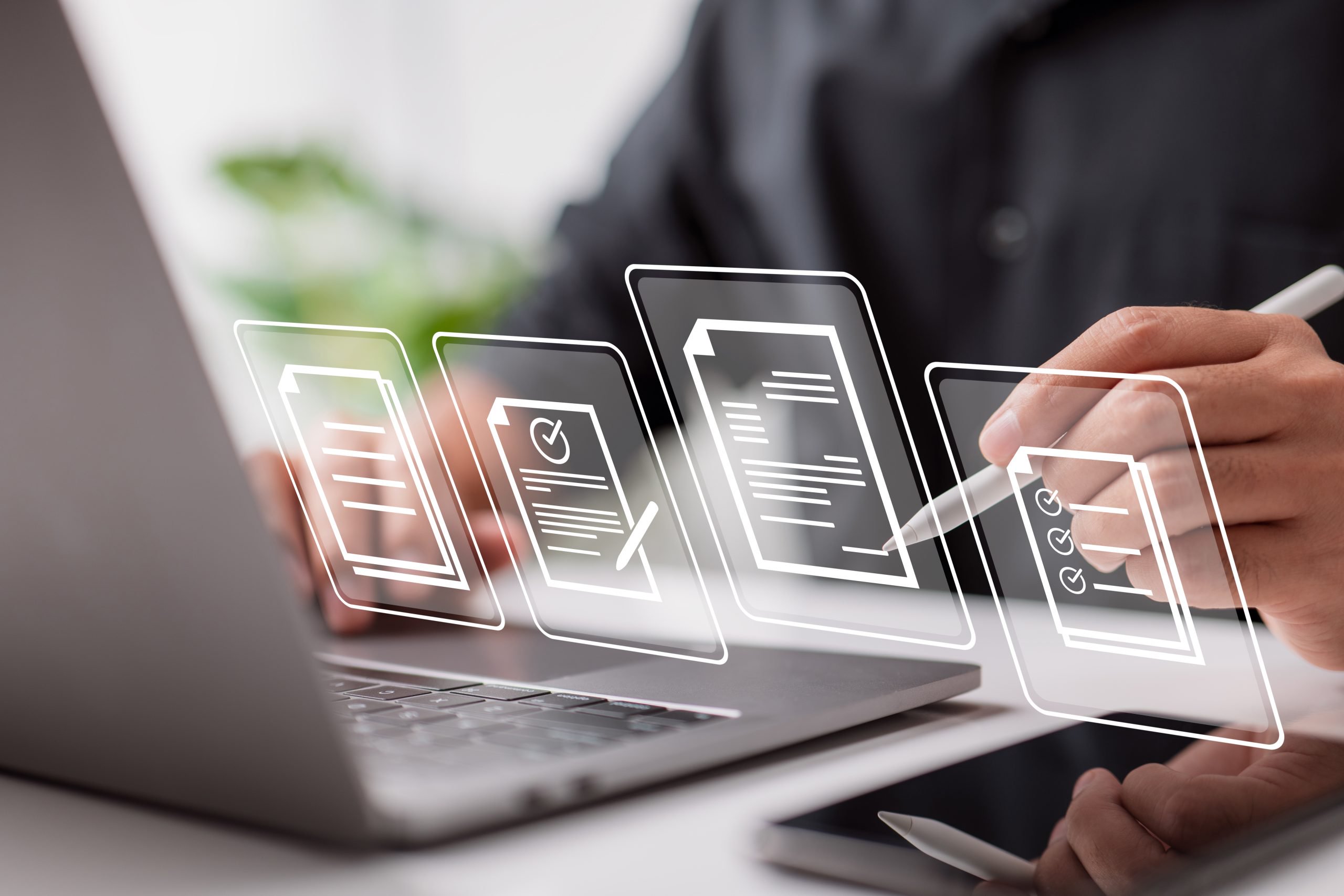
Twitter users urged to trigger SARs against energy...
Big Data & AnalyticsDigital TransformationInnovation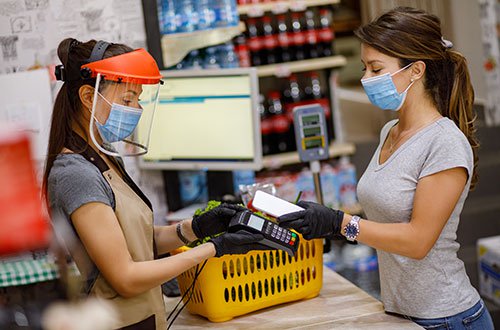
Retail After COVID-19: How Innovation is Powering the...
Digital TransformationInsightsConsumer and RetailTop Insights Categories

Let’s Work Together
Related Content
The Rise of The Invisible Bank
Banks will power experiences, but everyone will ignore them. Inspiration for this blog title comes from Jerry Neumann, the author of the blog Reaction Wheel, who wrote in 2015 that ‘software eats the world and everybody ignores it’. Neumann also observed that ‘information and communications technology becomes ubiquitous but invisible’ – in other words, … Continue reading Maximise your investment in Machine Learning →
Learn More
MLOps Principles Part Two: Model Bias and Fairness
Welcome back to the second instalment of our two-part series – MLOps (Machine Learning Operations) Principles. If you missed part one, which focused on the importance of model monitoring, it can be found here. This blog explores the various forms that model bias can take, whilst delving into the challenges of detecting and mitigating bias, … Continue reading Maximise your investment in Machine Learning →
Learn More
How Smart Cars Will Change Cityscapes
Which technologies do we need to improve the quality of life for people in the city and make governance more effective? What role does a car play in the city of the future, and how can it make traffic safer? GlobalLogic’s engineers are already working on solving these issues.
Learn More
Share this page:
-
-
-
-
URL copied!