
-
-
-
-
URL copied!
Modern enterprises spend a large amount of time and resources building data pipelines into the data platform from a variety of sources and managing the quality of data transferred through the pipelines. These pipelines can vary in terms of source systems, sink systems, transformations, and validations performed.
A pipeline created for a particular use case may not be reusable for a different one and will require additional development effort to change. As a result, there is a need for frameworks that build new pipelines, adding additional data sources or data sinks with minimal time and development effort. Ideally, the framework should also be flexible in customizing and extending it to easily adapt to suit enterprise-specific requirements.
A number of low code and no-code solutions exist that allow for visually creating the data pipelines across a variety of sources and sinks. However, they do not provide the flexibility and modularity typically required to customize the pipelines for a given scenario.
Using a low code framework consisting of reusable, modular components that can be stitched together to compose the required pipelines is a better approach.
In this post, you’ll learn about the requirements for the low code framework and the approach to designing this framework.
Requirements for the Framework
Creating and maintaining pipelines to move data in and out of the platform is a major consideration. A data platform framework that allows its users to perform the different operations in a consistent way, irrespective of the underlying technology, will greatly reduce time and effort.
What do you look for in a low code framework? Here are some suggested requirements.
Modular: The framework should be modular in design. Each component of the framework can be used, managed, and enhanced independently.
Out-of-the-Box Functionality: Support integration with common data sources and sinks, and perform transformations out of the box. The components should be easy to implement for common use cases.
Flexible: The framework should be able to integrate with different services/systems across clouds or from on-premises.
Extensible: Allow extending existing components to customize as per specific requirements or add new custom components to implement new functionalities.
Code First: Provide a programmable way of defining and managing pipelines. API and/or SDK support should be available to programmatically create and access the pipelines.
Cross Cloud Support: Support for data sources, sinks, and services across different cloud services. You should be able to migrate pipelines using the framework for one cloud or on-premises to another cloud environment.
Reusable: Provides common reusable templates that allow for creating jobs in an easy way.
Scalable: Ability to scale workers dynamically or by configuration to handle high performance. The framework should automatically scale the underlying compute in response to changing workloads.
Managed Service: The framework should be deployable on a fully managed cloud service. Provisioning the infrastructure capacity, managing, configuring, and scaling the environment should be managed automatically. Minor version upgrades and patches are automatically updated and support is provided for major version updates.
GUI-based Definition: An intuitive GUI for creating and maintaining the data pipelines will be useful. The job runs and logs from execution should be accessible through a job monitoring and management portal.
Security: Out-of-the-box integration with an enterprise-level IAM tool for authentication and role-based access control.
A High-level Overview of the Framework
The data platform framework provides the base foundation upon which you can build specific accelerators or tools for data integration and data quality/validation use cases.
Blueprint
While designing the framework, it is important to consider the following points:
- Technology Choice: We recommend a cloud-first approach when it comes to technology. The core of the framework should be deployable on a cloud-managed service that is extensible, flexible, and programmatically manageable.
- Data Processing: Data processing should be based on massively parallel processing solutions that can easily scale as per the requirement in order to support large volumes.
- Orchestration: Scheduling and executing data pipelines requires a scalable and extensible orchestration solution. Go with a managed workflow service that provides a programmable framework, with out-of-box operators for integration, and also allows for adding custom operators as required.
- Component Library: Common data processing functionalities should be made available as components that can be used independently or in addition to other components.
- Pipeline Configuration: A custom DSL-based configuration definition allows for reusability of pipeline logic and provides a simple interface for defining the required steps for execution.
Building Blocks
Here are the building blocks for such a framework:
- Pipeline Template: A DAG template that supports pipeline orchestration for different scenarios. The template can be used to generate data pipelines programmatically during design time, based on user requirements.
- Job Template: A job execution template that supports processing the data using the component library as per user requirements. Common job flow patterns can be supported through built-in templates.
- Component Library: A suite of functionality code for supporting different processing use cases. It consists of components, factories, and utilities.
- Components: The base processing implementations that perform read/write on various data sources, apply transformations, run data validations, and execute utility tasks.
- Factory and Generators: Factory and Generator code helps in abstracting the implementation differences across different technologies.
Accelerate Your Own Data Journey
At GlobalLogic, we are working on a similar approach as part of the Data Platform Accelerator (DPA). Our DPA consists of a suite of micro-accelerators built on top of a platform framework based on cloud PaaS technologies.
We regularly work with our clients to help them with their data journeys. Share your needs with us using the contact form below and we are happy to discuss your next steps.
Top Insights
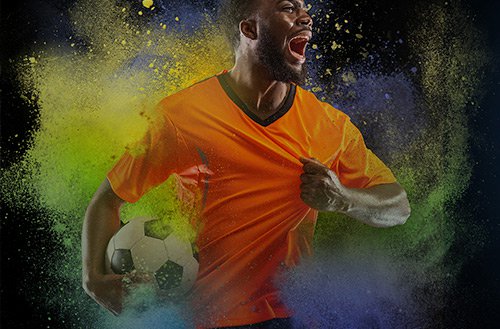
Manchester City Scores Big with GlobalLogic
AI and MLBig Data & AnalyticsCloudDigital TransformationExperience DesignMobilitySecurityMedia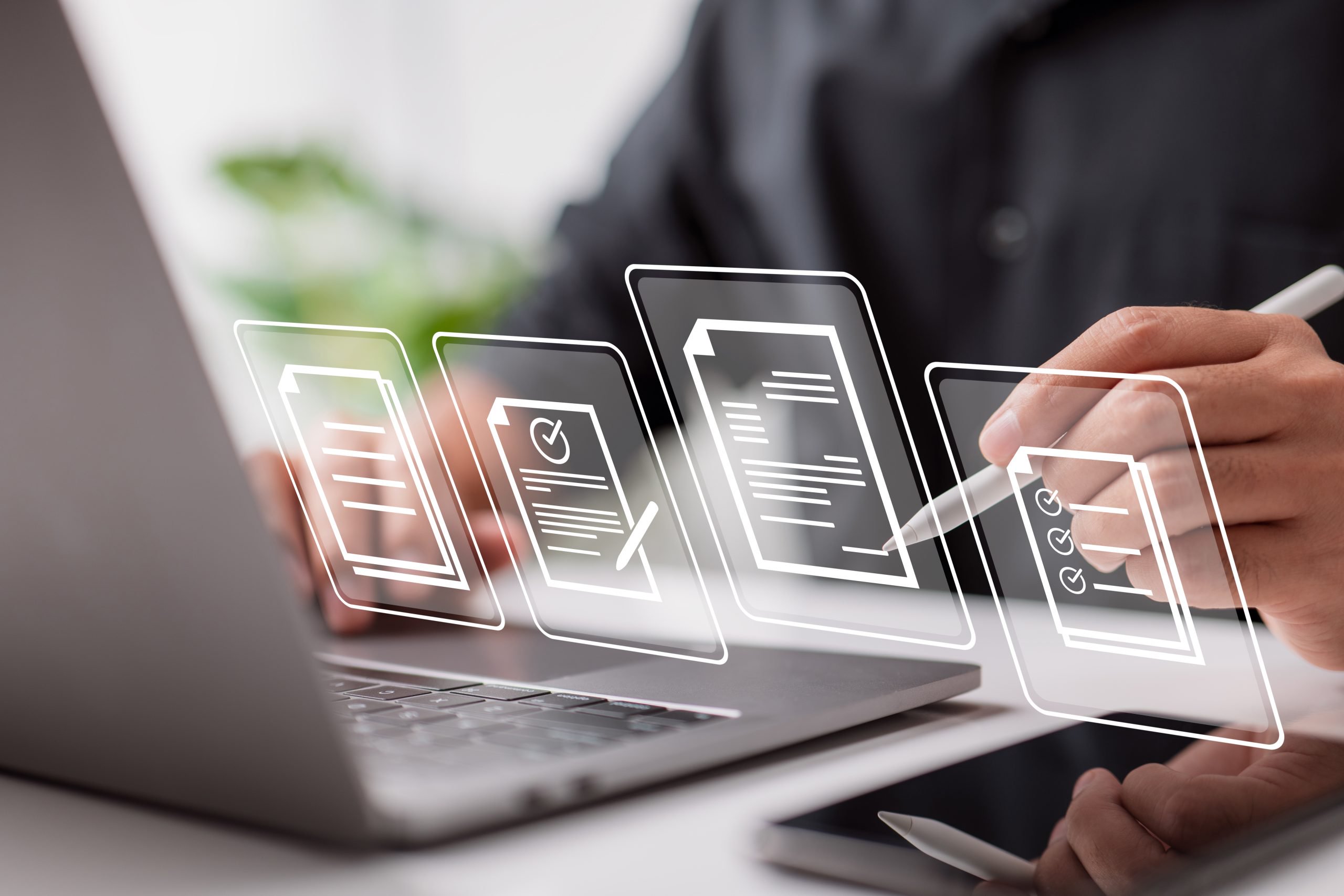
Twitter users urged to trigger SARs against energy...
Big Data & AnalyticsDigital TransformationInnovation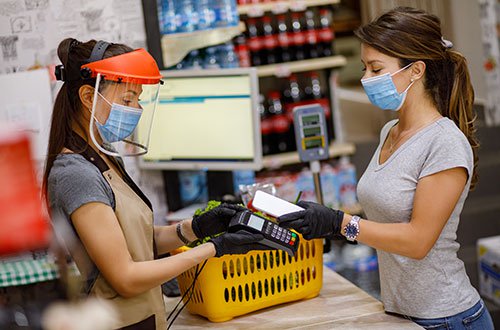
Retail After COVID-19: How Innovation is Powering the...
Digital TransformationInsightsConsumer and RetailTop Authors
Top Insights Categories

Let’s Work Together
Related Content
GlobalLogic wins at the 2023 Analytics Institute Awards, Dublin
*This blog was updated on Friday 16th June. The team is excited to announce that GlobalLogic was named winners of the Emerging Technology Award at last night's Analytics Institute Awards! This prestigious award recognises organisations that have successfully employed new technologies such as IoT, Edge Computing, Machine Learning, or RPA. Our submission showcased the successful application of … Continue reading Global Practices: Low Code Composable Data Platform Framework →
Learn More
MLOps Principles Part Two: Model Bias and Fairness
Welcome back to the second instalment of our two-part series – MLOps (Machine Learning Operations) Principles. If you missed part one, which focused on the importance of model monitoring, it can be found here. This blog explores the various forms that model bias can take, whilst delving into the challenges of detecting and mitigating bias, … Continue reading Global Practices: Low Code Composable Data Platform Framework →
Learn More
The GlobalLogic Academy Programme – a personal, introspective recollection
Ben Graham – Academy 2022 Graduate/Delivery Consultant I am currently in the DevOps capability for consulting and a recent graduate of the Academy 2022 programme which ran from September to December. I’d like to detail my thoughts on the process and share how my fellow graduates and I felt going on this journey. The GlobalLogic … Continue reading Global Practices: Low Code Composable Data Platform Framework →
Learn More
Seven steps to break down systemic gender barriers
Despite progress over the years, women are still significantly underrepresented in tech. Systemic gender barriers – such as unconscious bias, lack of access to education, and cultural norms – can make it difficult for women to break into the tech industry. But how do we break down these barriers? Follow our simple step by step … Continue reading Global Practices: Low Code Composable Data Platform Framework →
Learn More
MLOps Principles Part One: Model Monitoring
Machine learning (ML) has quickly become one of the most transformative technologies of our time – with applications in a wide range of industries, from healthcare and finance to retail and transportation. As organisations begin to adopt ML, they are facing new challenges arising from working with ML systems. Building, deploying and maintaining ML models … Continue reading Global Practices: Low Code Composable Data Platform Framework →
Learn More
Share this page:
-
-
-
-
URL copied!