
-
-
-
-
URL copied!
Digital product development can be a game-changer for organizations, in the ways it facilitates a seamless, software-driven user experience. It can provide insights on taking a user-centric approach to planning and developing digitally-driven solutions that delight users, create new lines of revenue, and scale with your growing business.
Consistently applying a data-driven approach to digital product development helps your organization uncover customer insights, identify market trends, and validate hypotheses that result in products that better meet customer needs and drive business growth. Moreover, continuously iterating based on real-time insights ensures the products you've invested in are sustainable and evolve with your customers' needs.
In today’s world, organizations are accumulating and sitting on large volumes of data from an increasing number of systems and interfaces. However, this comes with its fair share of challenges, including (but not limited to) data quality and reliability, scalability and infrastructure, data privacy and security, and the growing talent and expertise gap. We’ll take a closer look at these key considerations and more, so you can achieve a more data-driven approach to digital product development.
1. Data Quality, Reliability & Governance
While the availability of vast amounts of data offers opportunities for valuable insights, it also introduces the risk of incomplete, inaccurate, or inconsistent data. Ensuring data quality and reliability is essential to leveraging the full potential of a data-driven approach.
Incomplete or missing data can result in incomplete or skewed insights, leading to flawed decision-making. Without reliable data, organizations risk basing their strategies on faulty assumptions or incomplete information.
Overcoming this challenge calls for robust data governance processes. This includes defining data standards, establishing data collection and storage protocols, and implementing quality checks. Data validation techniques, such as data profiling, outlier detection, and consistency checks, are crucial in identifying and rectifying data anomalies. Regular data audits and monitoring processes help maintain data integrity and reliability over time.
Additionally, organizations can employ automated data validation tools and techniques to streamline the process and ensure a higher level of data quality. These tools can flag data inconsistencies, identify missing values, and validate data against predefined rules or business requirements.
2. Scalability and Infrastructure
The ability to process and analyze large volumes of data is essential for effective digital product development. As organizations gather increasing amounts of data from diverse sources, scalability and infrastructure become critical factors in harnessing the full potential of this data.
Traditional systems and infrastructure may not be equipped to handle the velocity, variety, and volume of data that digital product development demands. Processing and analyzing massive datasets require powerful computing resources, storage capacity, and efficient data processing frameworks.
Investing in scalable infrastructure ensures organizations can handle ever-growing data volumes without compromising performance. Cloud-based solutions, such as scalable cloud computing platforms and storage services, offer the flexibility to scale resources up or down based on demand. This elasticity allows organizations to handle peak workloads during intense data processing and analysis periods while avoiding excessive costs during periods of lower activity.
Modern technologies like distributed computing frameworks, such as Apache Hadoop and Apache Spark, provide the ability to parallelize data processing across clusters of machines, improving processing speed and efficiency. These frameworks enable organizations to leverage distributed computing power to tackle complex data analytics tasks effectively.
Recommended reading: The Evolution of Data & Analytics Technologies
3. Data Privacy and Security
A strong focus on data privacy and security in digital product development helps organizations maintain compliance, protect sensitive data, and foster customer trust. This, in turn, allows for more effective data-driven decision-making and enables organizations to leverage the full potential of their data assets while mitigating the inherent risks.
It's not a matter of if it will happen but when, as IBM reports that 83% of organizations will experience a data breach. Those using AI and automation had a 74-day shorter breach lifecycle and saved an average of USD 3 million more than those without.
Safeguarding customer information and maintaining trust is crucial in a data-driven approach. This data often includes sensitive and personal information about individuals, such as personally identifiable information (PII) or financial data. Protecting this data from unauthorized access, breaches, or misuse is of paramount importance.
Organizations must comply with data privacy regulations, such as the General Data Protection Regulation (GDPR) or the California Consumer Privacy Act (CCPA). These regulations outline guidelines and requirements for the collection, storage, processing, and sharing of personal data. Adhering to these regulations ensures that organizations handle customer data responsibly and legally.
Companies can implement encryption techniques to protect data at rest and in transit, access controls, and user authentication mechanisms. Conducting regular security audits and vulnerability assessments is also best practice. Supporting these initiatives requires a culture of data privacy and security awareness among employees. Training programs and clear communication channels can help employees understand their roles and responsibilities in protecting data and recognizing potential security risks.
4. Interpreting and Extracting Insights
Extracting meaningful insights from complex and diverse datasets is crucial for driving product innovation and success. However, this task can be challenging without the expertise of skilled data scientists and analysts to apply advanced analytical techniques and statistical models. These professionals possess the skills to navigate vast amounts of data, identify relevant patterns, and extract actionable insights that inform product development strategies.
Data scientists and analysts involved in digital product development must have a deep understanding of statistical analysis, data mining, machine learning, and visualization techniques. They should also possess domain-specific knowledge to contextualize the data and derive meaningful insights relevant to the product and its target audience.
These professionals leverage analytical tools and programming languages to manipulate and analyze data, such as Python, R, SQL, and data visualization tools like Tableau or Power BI. They employ exploratory data analysis techniques, statistical modeling, predictive analytics, and other advanced analytical methods to uncover patterns, correlations, and trends within the data.
They can identify user behavior patterns, preferences, and pain points, allowing organizations to make data-driven decisions about feature enhancements, user experience improvements, and product roadmaps. Collaboration between data scientists, analysts, and product development teams is crucial for the successful interpretation and application of data insights.
And, of course, this leads us to...
5. Talent and Expertise Gap
Successfully blending software engineering and data analytics expertise enables organizations to build data-driven products that offer exceptional user experiences. However, bridging the talent and expertise gap by finding skilled professionals with a strong understanding of both disciplines can be a significant challenge.
Software engineers possess the technical prowess to design and build robust and scalable applications, while data analytics professionals can extract meaningful insights from data and apply them to inform product development strategies. The intersection of these skill sets is relatively new, and the demand for professionals who can bridge the gap is high. This creates a talent shortage and a competitive job market for individuals with software engineering and data analytics expertise.
To address this challenge, organizations must invest in talent acquisition strategies that attract individuals with hybrid skill sets. They can collaborate with educational institutions to develop specialized programs that equip students with the necessary knowledge and skills in both domains. Providing internships, training programs, and mentorship opportunities can also help nurture talent and bridge the expertise gap.
Organizations can foster cross-functional collaboration to encourage knowledge sharing between software engineering and data analytics teams. This allows professionals from different disciplines to learn from each other and leverage their collective expertise to drive innovation in digital product development.
Additionally, promoting a culture of continuous learning and professional development is crucial. According to McKinsey, which takes regular pulse checks of product-development senior executives, 53% of decision-makers believe skill building is the most useful way to address capability gaps, ahead of hiring, talent redeployment, and contracting in skilled workers. Encouraging employees to enhance their skills through training programs, industry certifications, and participation in conferences and workshops helps keep them updated with the latest advancements in software engineering and data analytics.
Recommended reading: A Digital Product Engineering Guide for Businesses
6. Data Integration and Compatibility
Integrating and compatibility between disparate data sources and systems is a major challenge for organizations. Establishing seamless data integration pipelines and ensuring system compatibility is crucial for successful data-driven digital product development.
Organizations often have many data sources, including internal databases, third-party APIs, customer feedback platforms, social media platforms, and more. These sources can generate data in various formats, structures, and locations, making integrating and harmonizing the data effectively complex.
Legacy systems further compound the challenge. Older systems may have limited compatibility with modern data analytics tools and techniques. Extracting, transforming, and loading data from legacy systems for analysis can be cumbersome and time-consuming.
To address these challenges, organizations need to adopt a strategic approach to data integration, including:
- Data architecture and planning to develop a robust data architecture that outlines data flows, integration points, and data transformation processes. This architecture should account for different data sources, formats, and systems in the product development lifecycle.
- Data integration tools and technologies to simplify the integration of disparate data sources. These tools can help automate data extraction, transformation, and loading (ETL) processes, ensuring smooth data flow across systems.
- API and middleware integration, which can facilitate seamless integration between systems and data sources. APIs provide standardized interfaces for data exchange, allowing different systems to communicate and share data effectively.
- Data transformation and standardization. Data transformation techniques play a vital role in harmonizing data from different sources. Standardizing data formats, resolving inconsistencies, and ensuring data quality during the transformation process enables more accurate and reliable analysis.
- Modernization efforts to improve compatibility with data analytics tools and techniques. This digital transformation could involve system upgrades, adopting cloud-based solutions, or implementing data virtualization approaches.
7. Data Visualization and Communication
By using data visually to tell a story through charts, graphs, dashboards, and other interactive visual elements, organizations can distill complex information into intuitive and easy-to-digest formats. Data visualization is pivotal in effectively communicating complex data insights to non-technical stakeholders.
In its raw form, data can be overwhelming and difficult to comprehend for individuals without a technical background. Complex datasets, statistical analyses, and intricate patterns can easily get lost in rows of numbers or dense spreadsheets. This is where data visualization comes into play, allowing stakeholders to grasp the key insights and trends at a glance.
Effective data visualization relies on understanding the audience and tailoring the visual representations accordingly. Different stakeholders have varying levels of familiarity with data and different areas of interest. The visualizations should be designed to align with their needs, ensuring the right information is conveyed clearly and concisely.
There are several key principles to consider when designing data visualizations for effective communication, including simplifying complex data, a visual hierarchy that highlights important information, contextualization and relevant comparisons, interactivity, and compelling storytelling.
Recommended reading: 4 Best Practices to Guide IoT and Dashboarding Projects
8. Ethical Use of Data
The collection and analysis of vast amounts of data give rise to ethical considerations. As organizations harness the power of data to drive product development strategies, it is essential to uphold the highest standards of ethical conduct. This includes respecting user privacy, protecting sensitive information, and ensuring data usage complies with applicable laws and regulations.
Obtaining informed consent from users is essential. Organizations must be transparent about the data they collect, how it is used, and the measures in place to protect it.
Fairness is another crucial aspect of ethical data use, ensuring that the organization is using unbiased algorithms, models, and analytical techniques that do not discriminate against individuals or perpetuate societal biases. Proactively assess and mitigate potential biases in data collection, analysis, and decision-making processes to ensure fairness and equity.
Social responsibility is another guiding principle in data-driven product development. Advocate for the ethical use of data to address societal challenges, foster positive social impact, and avoid harm to individuals or communities. Consider the broader implications of data practices and determine how your organization can actively contribute to creating a responsible and inclusive digital ecosystem.
Implementing ethical data practices requires a comprehensive approach that includes clear policies, regular audits, and ongoing training for employees. It's well worth getting right. Ethical data practices contribute to the long-term sustainability and reputation of organizations, while also aligning with broader societal expectations and regulatory requirements.
9. Cost and ROI
Implementing big data and analytics solutions in digital product development comes with significant upfront costs, including investments in infrastructure, tools, and talent acquisition. Organizations must carefully evaluate the return on investment (ROI) to ensure that the benefits derived from analytics initiatives outweigh the associated expenses.
While the costs of implementing big data and analytics solutions can be substantial, the potential benefits are equally significant. Leveraging data efficiently allows organizations to gain valuable insights, make informed decisions, and drive business growth. Research from The Business Application Research Center (BARC) shows that companies leveraging their data efficiently see an average increase in profitability of 8% and a 10% reduction in costs.
Begin by clearly defining the specific business objectives and key performance indicators (KPIs) your big data and analytics initiatives aim to address. This provides a basis for evaluating the impact and effectiveness of the investments made.
Conduct a thorough cost-benefit analysis to assess the potential returns and associated costs of implementing big data and analytics solutions. Consider both tangible and intangible benefits, such as improved decision-making, enhanced customer experience, and increased operational efficiency.
When investing in infrastructure, consider scalability to accommodate future growth and increasing data volumes. Cloud-based solutions offer the flexibility to scale resources based on demand, minimizing upfront infrastructure costs while providing the necessary capabilities to handle growing data requirements.
Establish mechanisms to measure and track the ROI of big data and analytics initiatives. You'll need to regularly assess the impact on key business metrics, such as revenue growth, cost savings, customer satisfaction, and operational efficiency.
10. Continuous Learning and Adaptation
Staying current with the latest advancements, best practices, and industry trends is vital in digital product development, where technological advancements, new methodologies, and emerging opportunities drive constant evolution. To remain competitive and harness the full potential of data, thought leaders must foster a culture of continuous learning and adaptability within their organizations.
Encourage teams to pursue professional development opportunities. It's important to allocate time and resources for training and learning activities and provide access to relevant educational resources to facilitate these programs. Give employees space and time to establish knowledge-sharing platforms and communities of practice to facilitate the exchange of ideas and encourage collaboration, as well.
Agile methodologies, such as Scrum or Kanban, are great for promoting iterative development and continuous improvement. Apply these methodologies to data analytics projects to enable teams to adapt quickly to changing requirements, incorporate feedback, and continuously learn from data insights and even failures.
Continuous learning should extend beyond the boundaries of data and analytics, as cross-disciplinary collaboration and combining data-driven insights with domain expertise can lead to more innovative approaches in digital product development. Developing data literacy across the organization is crucial, and empowers individuals to make informed decisions, contribute to data-driven discussions, and effectively communicate insights to drive organizational success. Advocate for understanding and interpreting data among all stakeholders, regardless of their roles or technical backgrounds.
Conclusion
Applying a big data and analytics lens to digital product development means taking a strategic, data-driven approach encompassing technical solutions, organizational cultural shifts, investment in talent and infrastructure, adherence to ethical principles, and a culture of continuous learning.
Yes, it's a tall order. Working alongside an experienced digital engineering partner like GlobalLogic through ideation, design, development, testing, deployment, and ongoing maintenance can help. We help organizations unlock the true potential of their data and get to market faster with innovative, compliant digital products that drive business success.
Want to learn more? Contact the GlobalLogic team today and see what we can do for you.
Trending Insights
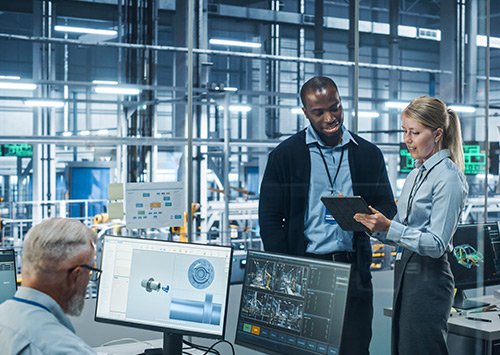
If You Build Products, You Should Be Using...
Digital TransformationTesting and QAManufacturing and Industrial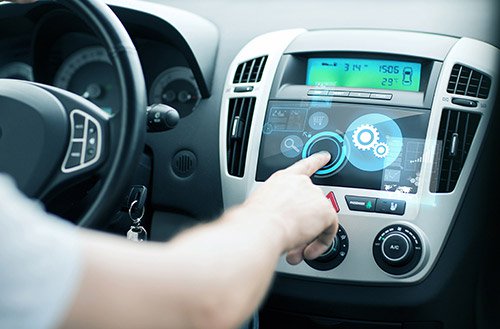
Empowering Teams with Agile Product-Oriented Delivery, Step By...
AgileProject ManagementAutomotiveCommunicationsConsumer and RetailMedia
Let’s Work Together
Related Content
Unlock the Power of the Intelligent Healthcare Ecosystem
Welcome to the future of healthcare The healthcare industry is on the cusp of a revolutionary transformation. As we move beyond digital connectivity and data integration, the next decade will be defined by the emergence of the Intelligent Healthcare Ecosystem. This is more than a technological shift—it's a fundamental change in how we deliver, experience, … Continue reading 10 Ways Big Data & Analytics Supports Digital Product Development Success →
Learn More
Crowd-Striked: Lessons Learned and Best Practices for Future Prevention
Incident Summary On July 19, 2024, CrowdStrike released a content configuration update for the Windows sensor that resulted in widespread system instability, causing Windows systems to experience the "Blue Screen of Death" (BSOD). The issue was traced to a channel file named “C-00000291*.sys” included in the update, which caused system crashes upon deployment. “Channel files” … Continue reading 10 Ways Big Data & Analytics Supports Digital Product Development Success →
Learn More
Share this page:
-
-
-
-
URL copied!