
-
-
-
-
URL copied!
Dark data remains hidden in the form of unstructured emails, social media posts, and digital and scanned documents including invoices, purchase orders, contracts, written agreements and policies, survey results, and application forms.
How can your organization derive value and reap benefits from the dark data using advanced analytics and techniques like AI, Deep Learning, and NLP? In this post, we explore the benefits and challenges of activating dark data and share examples of how dark data is delivering meaningful value.
What is Dark Data?
Gartner defines dark data as, “The information assets organizations collect, process, and store during regular business activities, but generally fail to use for other purposes (for example, analytics, business relationships and direct monetizing).Similar to dark matter in physics, dark data often comprises a universe of information assets. Thus, organizations often retain dark data for compliance purposes only. Storing and securing data typically incurs more expense (and sometimes greater risk) than value.”
According to industry reports, a large portion of unstructured data is never analyzed. There are two key reasons why:
- There is simply too much dark data to analyze
The sheer amount of data generated these days makes activating its insights difficult. The variety of data being collected can also be overwhelming, as it ranges from social media data and IoT/sensors to data buried in documents, call records, and transcripts from audio and video files.
- We lack specialist tools for analyzing dark data.
The technology required for effectively deriving value from such data for practical production scenarios is limited.
AI Advancements Are Driving New Possibilities for Dark Data Analysis
Over the last 50 years, we have seen Moore’s Law—that the number of transistors on a microchip doubles every two years, though the cost of computers is halved—realized as computer storage and processing capabilities have become smaller, cheaper, and faster.
We have also witnessed many other technological advancements such as:
- The emergence of more distributed computing and storage solutions.
- Innovations in processing technologies including graphics processing units (GPUs) and tensor processing units(TPUs).
- A resurgence of artificial intelligence (AI) including machine learning, natural language processing (NLP), and deep learning.
These have all significantly contributed towards making the collection, storage, and analysis of all kinds of data possible.
AI and related technologies have been applied to the classification of written texts like spam in emails, the sentiment analysis of social media posts, the recommendations of most relevant news/media by search engines, and help assessing risks/threats to national security in social media posts.
Now, they are also being used as an effective way of identifying relevant information from both digital and handwritten documents for the purposes of:
- Automated extraction and storage of information in the form of key-value pairs.
- Three-way matching data from invoices, purchase orders, and receipts.
- Taking information from claim forms and application forms and passing it on to downstream systems.
- Processing more textual documents such as Statements of Work (SOWs), Master Service Agreements (MSAs), Tower Lease Agreements, International Bid Documents, SLAs, and policies enables organizations to extract various clauses and entities, automate downstream processing, and better assess risks.
Activating Dark Data is Not Without Its Challenges
Many organizations are still not utilizing dark data despite a general understanding and acceptance of the value gained from extracting information from it. Here’s why:
First, it is not at all easy to understand the semi-structured format of documents and artefacts such as purchase orders, invoices, forms, handwritten texts. Extracting data, meaning, and risks from long textual documents such as contracts, agreements, policies, etc. is inherently challenging. There are complications and complexities such as isolating specific documents from a cluster of documents, classifying them, and understanding sections, headers, tables, images, abbreviations, watermarks, stamps, bullet points, and much more.
It is important to give special consideration to the “tables” in the documents, as they are complex for machines to identify and comprehend.
Second, there are also issues associated with validating the extracted data for further processing. This must be automated to the possible extent and must be done before final approval. If all fields require manual validation before the final processing, the amount of savings and value that can be brought to the table is questionable.
Practical Solutions for Illuminating Dark Data
Similarly to how the human mind approaches document analysis (extraction, key-value pair identification, clause identification, risk analysis), solutions require an end-to-end amalgamation of technologies such as rule engine, traditional NLP, machine learning, deep learning, and cognitive search incorporating multiple pathways.
After extracting the required data and information, it must be filtered for manual validation only for low confidence extractions. The automation flow is further enhanced by allowing configurability of straight-through processing for extractions where confidence is higher.
Here are a few options for stitching the automation process:
- Manually validate only those fields which are low in confidence for accuracy
- Take a call at the document level and enable straight-through processing for all documents where overall confidence is high.
- This requires deeper analysis of the fields to be extracted and classification into categories of accuracy sensitivity on impact of high and low.
- For lower sensitivity fields, thresholds can be set lower; for high sensitivity, thresholds can be higher.
- Allowing settings to be completely configurable is key.
The real challenge (and excitement) is to make option #2 possible with an increasingly higher percentage of documents going via straight-through processing.
The whole concept of post-deployment model performance tracking, feedback mechanism, auto-learning new data, and improved model deployment after manual approval makes this a long-lasting, sustainable, and successful AI implementation for extracting value from the dark data.
Conclusion
“I am convinced that machines can and will think in our lifetime.” — Oliver Selfridge (The Thinking Machines — 1961).
Hidden dark data translates to hidden risks, value, profits, efficiencies, savings and worse for enterprises and organizations. There is an immense potential to uncover insights from this data and deliver meaningful business value to the enterprise.
A few examples of areas where this has been applied successfully include:
- Procure to Pay: for information extraction and enhanced automation in three-way matching.
- Contract Management and Administration: for review assistance, improved compliance, and risk management.
- Claims Processing: for enhanced automation and improved effectiveness and efficiency.
- Customer Onboarding: for enhanced automation and improved effectiveness and efficiency.
- Policy Administration: for information extraction and improved customer service.
- Knowledge Management: for improved and meaningful knowledge discovery.
Thanks to technological advancements, the time is upon us to start out on the journey towards improved automation. This will allow for valuable insights to be gained from this otherwise hidden treasure trove that is dark data.
Top Insights
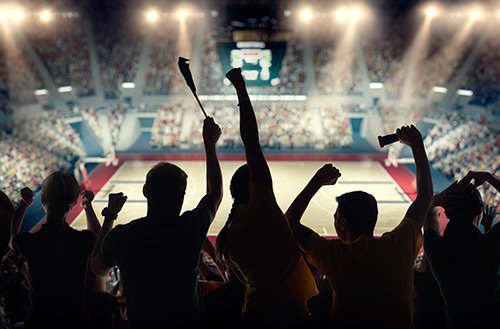
Best practices for selecting a software engineering partner
SecurityDigital TransformationDevOpsCloudMedia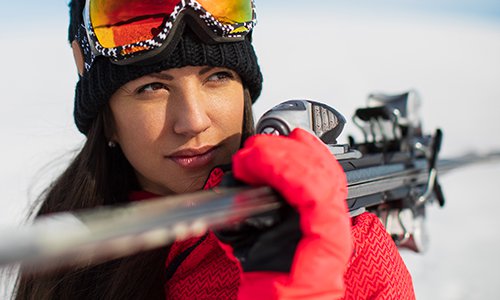
My Intro to the Amazing Partnership Between the...
Experience DesignPerspectiveCommunicationsMediaTechnology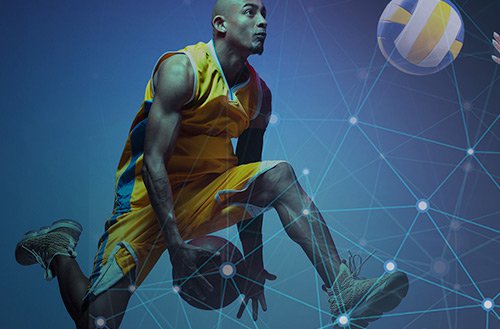
Adaptive and Intuitive Design: Disrupting Sports Broadcasting
Experience DesignSecurityMobilityDigital TransformationCloudBig Data & AnalyticsMedia
Let’s Work Together
Related Content
Unlock the Power of the Intelligent Healthcare Ecosystem
Welcome to the future of healthcare The healthcare industry is on the cusp of a revolutionary transformation. As we move beyond digital connectivity and data integration, the next decade will be defined by the emergence of the Intelligent Healthcare Ecosystem. This is more than a technological shift—it's a fundamental change in how we deliver, experience, … Continue reading Illuminating Insights Hidden in Dark Data →
Learn More
Power & Utilities – Changing Landscape
Power & utilities industry have gone through a supplied, and utilized, but it also poses threats to unparalleled shift in recent years, fueled by rapid established, legacy business models and regulatory technological advances, rising consumer demands, frameworks.
Learn More
Share this page:
-
-
-
-
URL copied!