
-
-
-
-
URL copied!
Several big data and analytic architectures, such as Lake House and Data Lake paradigms, are widely used by organizations to implement data and analytics platforms to uncover deeper insights into their data.
Newer architecture patterns are also evolving and have come to the foreground as organizations look for meaningful outcomes for their investments in big data, analytics, AI, and machine learning (ML) technology.
Among them, data fabric is one of the most interesting archetypes addressing data usage to incentivize businesses and help them extract value from their data.
With data fabric, it’s essential to leverage data and metadata to connect the dots and make it accessible across the organization. In this post, you’ll learn how a business can use a knowledge graph engine to power the data fabric architecture and help extract valuable data.
Data Fabric
Data fabric was defined by Forrester in 2016 as “a unified, integrated, and intelligent end-to-end data platform to support new and emerging use cases.”
Data fabric is an architectural concept that interweaves the integration of data pipelines and data assets to lay out a discoverable data landscape through automated systems and processes across environments. A data fabric doesn’t move data but provides an abstraction to make data available across the organization.
Below are the key components of the Data Fabric architecture:
- Data Cataloging
- Metadata Collection & Analysis
- Metadata Activation
- Data Integration
- Data Enrichment with Semantics through Knowledge Graphs
Knowledge Graphs
To unlock the value of data, you must understand all data formats, whether structured or unstructured. Even though data may be present in a single place, finding the hidden relationships and embedded knowledge for further analysis can still be challenging.
Having vast amounts of data has furthered the need for a representation of data that reflects a human understanding of the underlying information. To make information more easily digestible, data analysis needs to bring out relationships similar to the actual relationships in our world and not be bound by defined data schemas.
This is where knowledge graphs come into play. Knowledge graphs, an integral component in content engineering, can integrate billions of facts. Facts can come from disparate sources and formats, which can then be stored in graph databases and used for extracting further insights.
A knowledge graph represents a collection of connected descriptions of different entities, relationships, and semantic definitions. Entities can be actual objects, events, or even notional or abstract concepts.
Knowledge graphs combine database characteristics as the data can be queried, a graph as it forms a network, and a knowledge base as the data supports formal semantics. These combined characteristics can help interpret the data and help infer new insights.
The KG Engine of the Data Fabric
A data fabric relies on a set of tools and services to keep the components going and pull together the information of the fabric. For example, metadata can be collected as data is ingested or transformed and pushed to a metadata store or pulled directly from data stores or sources through data discovery tools.
Data is stored in various types of data stores post ingestion and processing. Data can also sit in different environments on-premises, on the cloud, or in multi-cloud and hybrid environments.
The Knowledge Graph (KG) Engine provides a mechanism to abstract the complexities related to data ingestion, data processing, and data storage and movement to provide a unified view of the data.
Below is a representation of the KG Engine setup to power the enterprise-wide data fabric:
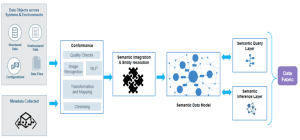
The metadata collected and the data available across systems and environments feed into a conformance layer for quality checks and extracting information in case the data is unstructured.
The conformed information undergoes entity resolution and semantic integration to create the semantic data models based on ontologies, which can then be questioned and inferred as part of the data fabric.
The KG Engine can be automated to ensure that the knowledge graph continuously evolves as more data sets and metadata becomes available. As a result, applications, algorithms, and teams can use the information.
In conclusion, knowledge graphs enable semantic data modeling and make it easier to understand the data. It also helps translate disparate data into information that can be consumed (through queries or visualization) for different decision-making purposes by organizational actors using the data fabric.
Learn more about activating the value of your organization’s data:
Trending Insights
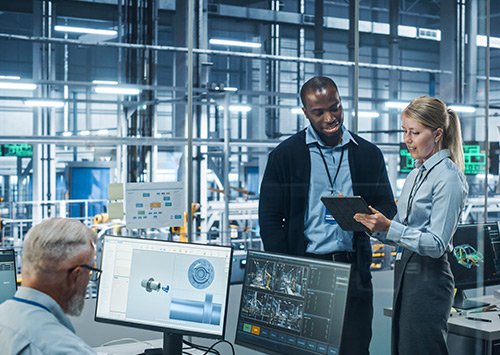
If You Build Products, You Should Be Using...
Digital TransformationTesting and QAManufacturing and Industrial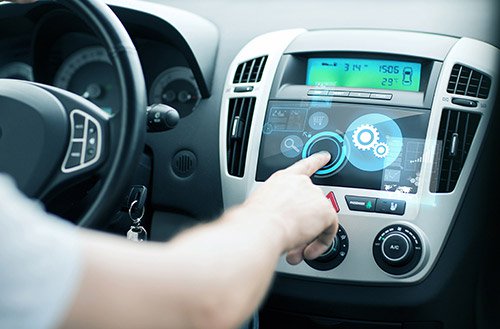
Empowering Teams with Agile Product-Oriented Delivery, Step By...
AgileProject ManagementAutomotiveCommunicationsConsumer and RetailMedia
Let’s Work Together
Related Content
Unlock the Power of the Intelligent Healthcare Ecosystem
Welcome to the future of healthcare The healthcare industry is on the cusp of a revolutionary transformation. As we move beyond digital connectivity and data integration, the next decade will be defined by the emergence of the Intelligent Healthcare Ecosystem. This is more than a technological shift—it's a fundamental change in how we deliver, experience, … Continue reading Powering the Data Fabric Architecture with a Knowledge Graph Engine →
Learn More
Share this page:
-
-
-
-
URL copied!